Architectural Frameworks for Big Data Analytics in Patient-Centric Healthcare Systems: Opportunities, Challenges, and Limitations
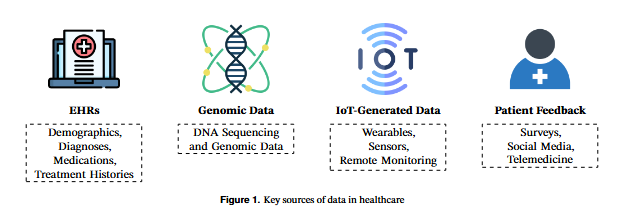
Published 2018-03-07
Keywords
- Big data analytics,
- Edge computing,
- Genomic data,
- Healthcare architectures,
- Machine learning
- Real-time analytics,
- Scalability ...More
How to Cite
Abstract
Patient-centered healthcare now relies heavily on big data analytics to provide personalized care and make informed decisions grounded in data. This research investigates the architectural frameworks that enable big data analytics in healthcare, with a focus on widely adopted systems such as Hadoop, Spark, cloud-based infrastructures, and hybrid models. These architectures handle diverse datasets, including Electronic Health Records (EHRs), genomic data, Internet of Things (IoT) device data, and patient feedback. This paper highlights their role in integrating, processing, and analyzing vast and complex data in real time. Challenges, such as data integration, scalability, real-time analytics, and privacy, are examined to identify limitations in existing frameworks. Key architectural concerns including heterogeneity of healthcare data and performance bottlenecks in real-time patient monitoring, are explored in depth. Analytical techniques and optimization methods are reviewed for their effectiveness in improving healthcare outcomes through predictive and prescriptive analytics. New possibilities for addressing current architectural limitations arise from emerging technologies like edge computing and federated learning, which offer low-latency processing and decentralized data analytics while safeguarding patient privacy. Enhancements to current architectures are proposed, with a focus on hybrid models that merge cloud and on-premises infrastructures, encryption techniques to protect sensitive patient data, and frameworks optimized for processing high-throughput genomic data and real-time analysis. These enhancements are intended to improve scalability, security, and real-time processing in order to enable more efficient and patient-centered healthcare systems.