Human-Centered AI (HCAI) Paradigms in Clinical Artificial Intelligence: An Analytical Discourse on Implementation Across AI Lifecycle Stages
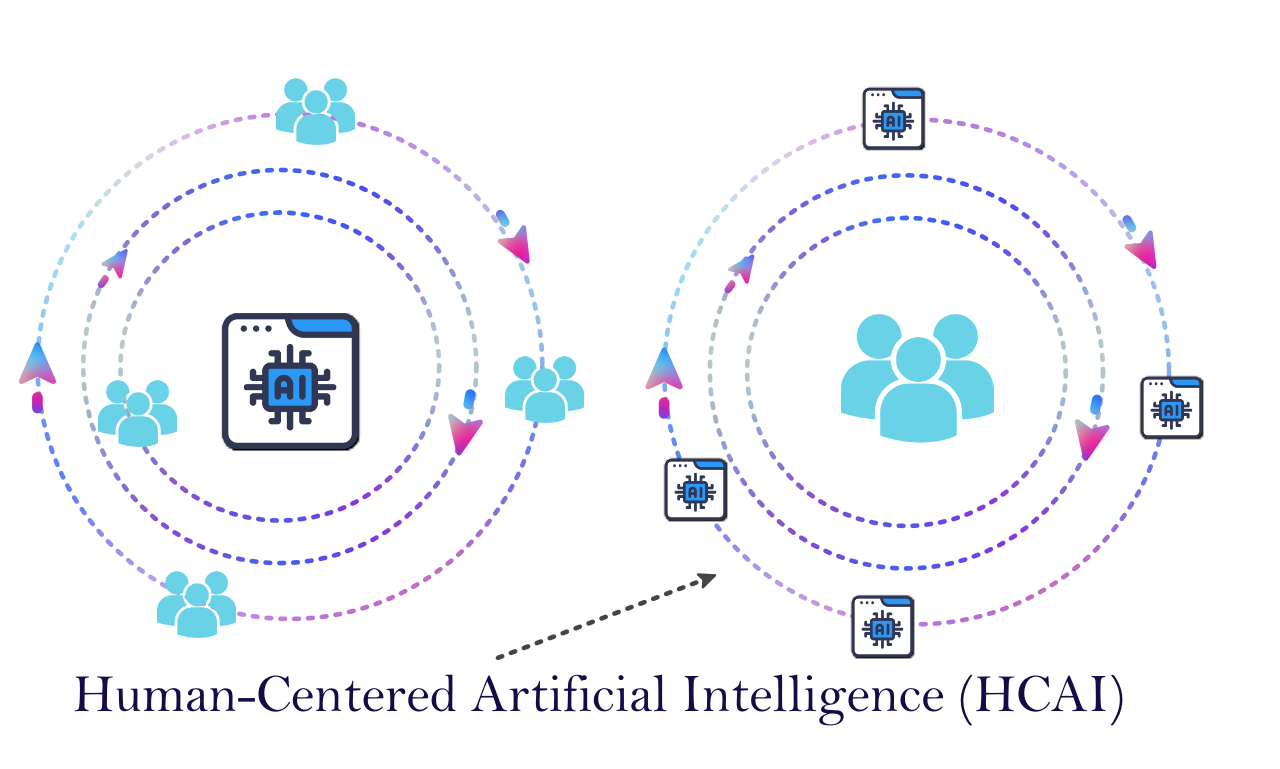
Published 2022-04-05
Keywords
- Clinical AI Algorithms,
- Data Acquisition,
- Ethical Design,
- Human-Centered AI (HCAI),
- Model Development
How to Cite
Abstract
Jatin Pal Singh
Abstract
Traditional discussions place humans around AI in decision-making, but the Human-Centered AI (HCAI) approach puts AI around humans, making humans the main focus. This paper presents an analysis of the key aspects of Human-Centered Artificial Intelligence (HCAI) in clinical AI algorithms, examining its implementation throughout the AI lifecycle stages. The focus is on the past literature and practical strategies to embed HCAI principles in each stage, namely Data Creation, Data Acquisition, Model Development, Model Evaluation, and Model Deployment. The research delineates how these stages can be optimized to enhance human performance, support ethical design, and ensure AI solutions are aligned with human values and societal needs. This research argues that in the Data Creation phase, the emphasis lies on inclusive and diverse data collection, ensuring that the data reflects a broad spectrum of demographics. The involvement of clinicians and patients is critical in making the data both comprehensive and reflective of real-world clinical scenarios. Ethical data collection practices are underscored, focusing on transparent consent and privacy safeguards. The integrity and contextual richness of data are also highlighted, stressing the need for accurate, complete, and relevant data collection. The Data Acquisition phase demands adherence to legal and regulatory frameworks, ethical data sourcing, and transparency in the data acquisition processes. The acquisition of diverse and representative data, focusing on quality and relevance, and responsible data partnerships are essential. Special attention is given to sociodemographic factors. In Model Development, the paper advocates for a clear definition of the AI model's purpose and ethical data handling. The model should be transparent, explainable, and designed with human factors in mind. Robustness, generalizability testing, iterative development, compliance with regulatory standards, and addressing societal and ethical concerns are vital. The Model Evaluation phase calls for performance evaluation across diverse populations, integration assessment within clinical workflows, fairness and equity analysis, and user feedback incorporation. Additionally, the model's explainability, robustness, regulatory compliance, and longitudinal performance monitoring are emphasized. In the Model Deployment phase, continuous monitoring of real-world performance, facilitation of understanding among clinicians and patients, ensuring ethical use, adaptation to diverse clinical environments, maintaining data security, responding to feedback, assessing the impact on clinical practice, and compliance with evolving regulations and standards are essential. This paper argues and highlights that the successful implementation of HCAI in clinical AI algorithms requires considering not only technical and scientific aspects but also ethical, legal, and social dimensions.